This week, we dive into the twists and turns of artificial intelligence with Olivier Ezratty, expert in AI and quantum technologies, on the occasion of the publication of his e-book on the uses of AI. We summarize 742 pages in a few lines to find out everything about the latest developments in technology or use of AI. Don’t thank us!
Here is a challenge: could you summarize your ebook in a few sentences?
It’s a big challenge! My process is often to update my books. This year, I added about a hundred pages compared to the previous version, which takes stock of technological developments and uses around AI.
To really summarize, recent developments have mainly concerned technologies and processors, but we can also see notable progress in project management, especially in the preparation of training data. They help speed up machine learning projects.
What changes in AI have you seen since your last publication in 2019?
I have observed several types of developments :
- Technological developments : In the first place, there have been technological developments in artificial intelligence with a significant improvement in language processing tools thanks, in particular, to the release of GPT3 in July 2020, the variants of these neural networks, which have dramatically expanded the range of possibilities in terms of text processing and generation. There have been equivalent developments in terms of image processing.
- Processor evolutions : I have also noted a remarkable evolution of processors dedicated to AI applications. There have been a lot of announcements in 2020 in the processor market, notably on Intel with its acquisition of Habana at the end of 2019, Nvidia with its new A100 GPGPUs announced in May 2020, or even Graphcore and its MK2 processors
- Changes in use : Usages have only changed little. The scenarios are often the same since we are in the maturing phase of the market. We are therefore going to find the same applications: Machine Learning, Deep Learning, language processing, artificial vision, etc. Currently, AI players are mainly in the deployment phase of their solutions. On the other hand, the issues are more operational and mainly concern how to ensure data quality, avoid bias in training data and protect privacy.
We can also mention some interesting new additions to the ebook :
- AI in the sea : in the context of maritime transport, AI makes it possible to carry out more detailed analysis of needs and delivery times, to optimize journeys, and to detect anomalies. AI helps mariners better understand maritime and ocean dynamics. Robotization is also taking off in the maritime environment and makes certain ships completely autonomous, without the need to call on a crew.
- AI in the context of covid : the benefits of AI have been sporadic in many use cases, there has not been the great revolution expected from this side. Despite everything, many AI-based tools have made it possible to solve very pragmatic problems such as, for example, the therapeutic retargeting of drugs, helping to find vaccines or optimizing the logistics of hospital systems in certain countries.
You have identified two major limitations to AI, what are they? How to resolve these biases?
I noticed that an amalgam was made in terms of AI biases: many people think that artificial intelligence biases come from algorithms when in reality, these biases have their main roots in the training data themselves, which quite simply reflect the biases of our societies. The rules of the algorithms are all quite neutral, as a rule. In contrast, algorithms can be used to debit training databases by increasing the proportion of under-represented groups. For example, if we have a database that reproduces the biases of society (not enough women, not enough minorities, various socio-demographic biases, etc.), the algorithm can increase the proportion of categories deemed too weak by concern for fairness.
The challenge for companies that use classification and prediction tools is to detect company biases as early as possible to resolve data biases using rebalancing algorithms, or by changing the sample. of the training base. It’s a question of social science.
This is also why it is essential to focus this type of thinking on generative AI. The latter are found in visual or textual content, and are capable of generating content themselves (scripts, films, poems, texts, etc.). They don’t really invent anything since they always create information which is a reconstruction made from existing data, mainly of human origin. They are a mixture of existing data, no more, no less.
And how is it a problem?
This is a problem insofar as AI will automatically reproduce societal biases, which will be perpetually reproduced. Every day, AI systems are based on partial data or extrapolations, and therefore perform poorly. This is the stupidity of AI and it can have disastrous consequences on people’s lives.
If, for example, we consider a system trained to offer higher education courses depending on the existing one. A pupil X living in Neuilly would be more likely to succeed than a pupil Y living in the 93 department, regardless of school results, only because the AI considers that the place of residence is decisive in the school success. This is the famous confusion between correlation and causation.
A more concrete example that took place at the end of 2018 at Amazon concerns gender bias. Amazon had implemented a Deep Learning system to analyze candidates’ CVs, which selected profiles with as many words as possible corresponding to the job description while taking into account socio-demographic characteristics. This system had been put in place to recruit tech profiles, and women made up only 5% of the training database, which was automatically reflected in reality. Indeed, the algorithm tended to disadvantage women who applied for these types of positions despite having the same skills as men. There was therefore a bias resulting from the training data. This can be integrated and corrected by Data Scientists taking into account the probabilistic mechanisms put in place by Machine Learning systems.
The solution for this kind of model is to overweight the under-represented category, or increase the percentage share of that category in the training base. It would be about doing positive discrimination.
Of all the forms of AI you mentioned (evolutionary algorithms, knowledge representation, affective AI, transfer learning), which is the most complex form, and therefore the most recent? How does it work in practice?
I’m still struggling to fully understand how the newer language processing algorithms, transformers, work. These are deep learning neural network systems capable of implementing what is called compositionality, that is, of processing text with a very good understanding of its context. It is a revolutionary solution for translating, classifying or generating text from an existing one. Even if these systems remain in a probabilistic approach to the text, the progress is significant. However, these applications are still artisanal in the sense that they assemble several interconnected bricks. It is still difficult to explain the results. So it’s quite difficult to grasp especially as it evolves very quickly. Indeed, each year, there are more than fifty new neural networks – of the Deep Learning type – which appear.
However, these systems still have serious limitations. For example, the German-born online deep learning translation service produces beautiful, grammatically correct translations from French to English. But the resulting English is not idiomatic. You can see from afar that it is French translated into English and not an English-native phrasing.
Basic Machine Learning working on structured data does not have this component of mystery: it is more coded, more segmented, it is based on better known and older mathematical models, which makes it easier to understand and explain the results.
How will AI evolve over the next few years?
In my opinion, two waves of AI are brewing: one aims to correct the flaws of current AIs, the other will be based on functionally improved AIs.
This first wave of corrective AI is already underway. We will find there the notion of frugal AI, because we know that AI consumes a lot of energy, especially in objects. Much research is underway to reduce the size of training games and ensure that less data is used for the same result. Other work relies on the quantization mechanism to reduce computational time when we use trained AI. This mechanism is based on the manipulation of whole numbers rather than floating point numbers. Finally, improved processors will also optimize power consumption.
The second element of the corrected AI relates to data biases that remain to be resolved. The third element concerns the protection of privacy. A technique promoted by Google in 2017 has spread widely in 2019 and 2020, called Federated Learning, and involves having an AI react to situations related to personal data to train a central AI, but without that personal data is sent to Google. A very widespread concrete example is provided by Google with its function of pre-filling emails for users.
The second wave will be a wave of improved AI, as opposed to corrective AI. In this context, many startups and developers are working on the mythical AI known as AGI (artificial general intelligence). It is an artificial intelligence which would reason generally like humans, and would therefore be able to solve any problem in the same way, if not better. This is a myth because I’m not sure you can reproduce this technically. Moreover, the experts working on the subject do not really define the uses that could be made of it.
However, we will be surprised in the next few years when it comes to image and language processing topics, because indeed innovators have yet to give their best. Many innovations are to be expected, especially in terms of use: the idea is not so much to revolutionize techniques, but above all to know how to assemble them to improve existing uses. This is why AI image analysis will be able to help us in particular in the research sector or AI that will help us in sharing knowledge. Indeed, many tools do not yet use AI in knowledge sharing. For example, today, when we ask Google a question, Google responds with URLs, but it would be a good idea for the tool to aggregate the information into one page. This would save time. Today this solution does not exist, and this gap is strongly linked to the way in which innovation works: on one side are the technologies, and on the other the uses, but it is sometimes difficult to mix them. If there is no economic logic in doing so, then there is little point in finding new solutions.
As I became interested in the history of computing, I was fascinated by how researchers worked on the creation of the first graphics computers and the mouse we use today. These researchers conceptualized things in the sixties, and it wasn’t until twenty years later that the material saw the light of day. It took eleven years of work between the first graphics computer created by Xerox in 1973 (the Alto) and the first Apple Macintoshes. Creative cycles are very long, but researchers and innovators have shown phenomenal creativity. This is why I think the same is true for artificial intelligence: innovators will find clever solutions that will revolutionize the uses of this technology.
What will the AI of 2030 look like? Should we consider new legislation? New forms of AI?
Yes, there will be legislation. Otherwise, we don’t know! How can we predict what people will be doing in 10 years?
One thing is certain, there will always be more data. But it is very difficult to predict how the algorithms will evolve. Elon Musk said “We were expecting flying cars, and we got Twitter”. There is always a gap between expectations and reality. It is very likely that many innovations will be in this scenario and rather unexpected and not necessarily that sophisticated from a technological point of view.
I would like to cite the example of GPT3, which is a language processing deep learning engine created by the American company OpenAI, initially launched by Elon Musk and largely funded by Microsoft more recently. This GPT3 is mainly driven by human intelligence since it contains all the data from Wikipedia, the Library of Congress, and a lot of data from websites. There are approximately 175 billion parameters in the neural network. GPT3 allows you to generate text and code. It’s truly amazing technology but it’s not yet embodied in the form of practical innovation!
At the same time, we have some totally dumb video conferencing software that doesn’t even detect open mics or active cameras when connecting to an online meeting. It is above all a question of the people behind the technologies. Some are creative while some go for the easy way. The various concerns that we may encounter are quite simply due to a lack of anticipation on the part of some developers.
The paradox is that AI is evolving in very sophisticated ways to answer ever more complex problems, but some basic problems still remain unresolved. This shows the gulf that there is between science and everyday use. I would like the two to come closer.
I also hope that the world of tomorrow will be a world where we can see people! Technology obviously allows us to stay in touch despite the context, but sometimes too much digital kills digital.
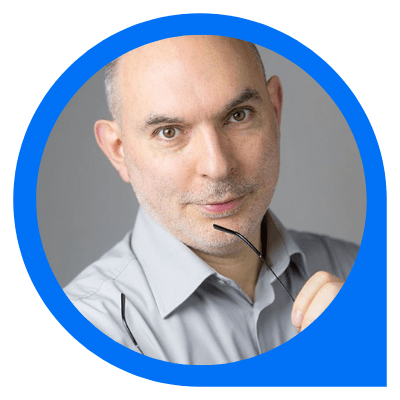
Olivier Ezratty advises companies on the adoption of deep techs in their innovation strategies, particularly around artificial intelligence and quantum computing. Author, speaker and trainer, he is notably the author of the ebook “The uses of artificial intelligence” (editions in 2016, 2017, 2018, 2019 and 2021) as well as “Understanding quantum computing” (editions in 2018, 2019 and 2020).
He is a trainer on artificial intelligence and quantum computing at Cap Gemini Institut, member of the Scientific Council of ARCEP as well as speaker and referent expert at IHEDN (class of 2019/2020).
Olivier Ezratty started in 1985 as a software engineer and R&D manager in editorial IT at Sogitec, a subsidiary of the Dassault group, then cut his teeth in marketing at Microsoft France to become Marketing and Communication Director and Relations Director. Developers (1990-2005). He is a Centrale Paris engineer (CentraleSupelec since 2015)