Only half of all AI projects have been deployed today. Why? Because it often takes longer than it looks – between 12 and 18 months. That is what comes out of the many studies of Gartner, Capgemini or BCG: the need to industrialize. A new emerging concept called DataOps was born from the complexity to deploy those data projects. Seen as the DevOps successor, it still differs due to the challenges you only come across when it comes to processing large amounts of data.
A bit of history about data projects
Data processing used to mean IT, and that was not so long ago. Big Data was not so big and analyzing it actually meant analyzing structured data. As for storage, it was basically databases or data warehouses that were fed with data.
Everything changed around 2010 with Data Lakes. Tools allowed us to look for data where it is and sources multiplied. Unstructured data (images, texts, audios…) could be processed, and in larger quantities. That’s what we now call Big Data.
Along with Data Lakes came Data Labs ; along with Data Labs came Data Scientists and Data Engineers. And here we are! All of these changes brought freedom to the teams but deployment remains a central issue as Analytics ecosystems don’t share the same IT delivery criteria as software development frameworks. Converting an idea into a POC becomes possible, but getting it deployed is still problematic.
The data project remaining issues
Infrastructure and security issues often come up, but we decided to talk about the ones that often cause the most problems and can be pretty hard to address:
The technological challenge: finding use cases is good, having the technologies to support them is better. The main problem here is that there isn’t a unique tool that allows to manage such a project. A lot of technologies are involved and you don’t only have to choose, you also need to make them all work together and follow their evolution as they need to be maintained and updated.
The human challenge: once you find the right technologies, you need to make it fit with the teams. Data Engineers prepare data, Data Scientists make it valuable. Their jobs are quite different and having them collaborate can be tough.
The process challenge: having the right tools with the right people to use them is still not enough. When it comes to data, you need processes implemented to make sure it all runs smoothly. Automation is key.
That’s when DataOps comes in. But as it is inspired by DevOps, let’s start with that.
What is DevOps?
DevOps is the portmanteau of “Development” and “Operations”. It is a technical, cultural and organizational approach that aims to accelerate features and applications delivery.
The main goal is to accelerate the “time-to-market” with shorter development cycles, more frequent deployments and continuous deliveries.
DevOps is based on two main concepts, Continuous Integration (CI) and Continuous Delivery (CD):
- Continuous Integration: it consists in building, integrating and testing new code in a repeated and automated way. It enables to quickly identify – and thus solve – potential issues.
- Continuous Deployment automates software delivery. Once an app has gone through every step of qualification testing, DevOps allows it to go to production.
To put it simply, the DevOps approach ensures the development team and the operations team alignment and allows to automate every step of the software creation cycle, from its development and deployment, to its management.
What is DataOps?
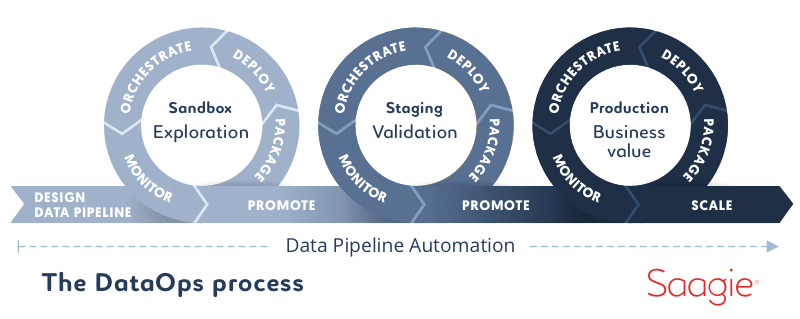
Gartner defines it as “a collaborative data management practice focused on improving the communication, integration and automation of data flows between data managers (Data Engineers, Data Architects, Data Stewards) and data consumers (Data Scientists, Business Analysts, Business teams) across an organization.”.
DataOps aims to improve and optimize Data & Analytics lifecycle in terms of rapidity and quality. DataOps uses technology to automate conception, deployment and management of data deliveries. It serves as a technological orchestrator for your project.
It shares with DevOps the goal to put collaboration at the heart of the project. The Agile Manifesto recommends: “people over process over tools”. Many people are involved in Data & Analytics projects. Making them all work together is at least as important as what will be your choice of technologies.
Their common principles and main distinctions
A few practices are common to DevOps and DataOps:
- Automation (CI/CD)
- Unit tests
- Environnements management
- Versions management
- Monitoring
These practices favor communication and collaboration between teams, allow quicker projects deployment and reduced costs.
The main goal of DataOps is to ease and accelerate delivery, and doing it while having new profiles (and data) involved in the process, managing different environments and orchestrating a lot of technologies. A game changer once you manage to properly apply it within the firm, and having the right tool might help you do it.
DataOps is a lot like DevOps, but it is harder to set up as it is applied to data and not just software development, which means both teams – Data and IT – don’t even work for the same department. In more specific terms, DevOps offers automation and agility but shows limitations when it comes to creating applications that are meant to process data in real time. And Data & Analytics projects mean building and maintaining data pipelines (or data flows).
A data pipeline represents a data flow, from its conception to its consumption.
Data comes from one end of the pipeline, goes through numerous preparing and processing steps to exit as models, reports and dashboards. This pipeline is the “Ops” aspect of data analysis.
Other differences come from Data Science projects specificities :
- Results repeatability
- Model performances monitoring as models can quickly change depending on the data you use
- Models exposition to users
DataOps is a new emerging concept without any truly defined standards or boundaries yet. But the results are already getting noticed as data projects are deployed quicker when DataOps is involved.
The solution: the DataOps platform
We often hear “data is the new oil” but data may be important, the key is what you use to make it valuable. What you need is a refinery, because people don’t want oil, they want gas.
Adrien Blind - VP Product & Technology at Saagie
What if the refinery was the combination of an approach – DataOps – and a tool. A tool we call a DataOps platform and has a precise list of features to help you:
Manage data from extraction to consumption – including storage, preparation, processing, visualization – and control the whole process with centralized logs and increased traceability;
Both ease and accelerate Data & Analytics projects deployment as all the technologies you need are gathered, updated and available. Saagie offers a managed infrastructure and orchestrates best-in-class open source and commercial data technologies;
Improve collaboration and communication within the company as every member of the team is involved and work on a unique centralized tool.
Why is it so valuable? Because having such a platform allows to manage processing data pipelines and deploy them in an automated and possibly scheduled way – all of that from one environment to another. It enables companies to use the most popular technologies to deliver and run data projects easily, quickly, and reliably.