This week, we are delighted to chat with Augustin Marty, CEO of Deepomatic. It is a software editor specializing in computer vision. How does computer vision support us in our daily tasks? Find out more here!
Tell us the story of Deepomatic
Deepomatic is a company that grew out of a company called Smile, which used to be an image search engine. Concretely, it is an engine that makes it possible to make the link between an inspiring image (advertising in the street, magazine, photo on social networks) and an e-commerce product.
Subsequently, we quickly implemented tools to easily and quickly create precise image recognition algorithms. These tools have enabled us to enter the second phase of Deepomatic’s life, and to very quickly develop our second business model, which is more business-to-business and major accounts oriented. We have packaged the tools on a single low code platform intended for professionals to carry out and maintain image recognition projects.
We conceptualized the platform five years ago, and we told ourselves at the time that there was a lack of a tool dedicated to people in the business that allowed us to build image recognition applications to empower our clients who industrialize image recognition projects. We now have 13 customers in production who are developing around 8 different use cases.
Our value proposition is as follows: to enable our customers to maintain their image recognition applications, to evolve their AI for very large scale production. We are currently working with Suez on the recognition of waste around the world, with Sanofi, or with Peugeot on the development of driving assistance features. The Deepomatic platform allowed users to take control of the application and evolve the AI according to customer needs.
From that moment, we decided to make choices on the typology of use cases that we wanted to offer, to better support clients in all the transformative dimensions of projects by speaking their language. Between putting an application into production and making it the tool of choice in the group, there is a passage to the level of maturity which requires that we understand the challenges of our customers. We were only interested in the use cases that we were already dealing with and which I will illustrate later.
What is Computer Vision? How does it work in practice?
Computer vision is based on learning by example (deep learning).
If we take the case of vehicle windshield damage detection, image recognition is done in two stages. Indeed, the goal of AI is not only to be able to detect the damage, but to derive information from it taking into account the context and therefore a solution.
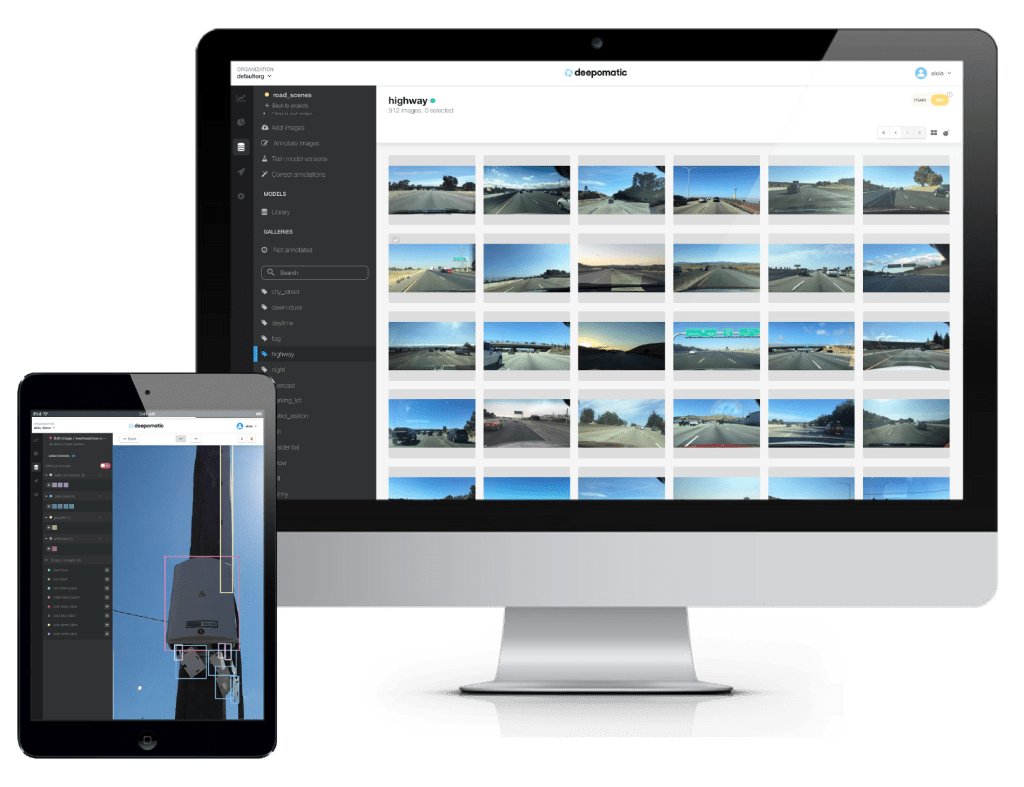
The first step in learning is to provide the coordinates of the damage in the image. This required pre-educating the detection neural networks through examples until they understood the information. So during the setup phase, you have to show the computer where the damage is. Then there are context rules (front, back, side, etc.). Finally, there is a set of business rules (depending on the country, the region) that allow the AI to derive suitable solutions.
In the second step, the AI must be able to diagnose the type of damage. It is only after receiving all the information mentioned above that the AI can be able to determine the solution to the problem (national rules, context, settings, etc.). The success of Deepomatic depends on its proper integration into the customer’s information system and on good control of the entire data lifecycle.
What is an augmented technician?
As I explained earlier, we have made choices about the use cases that we offer our customers. We therefore focused on “field services”. These are services that bring technicians and engineers to travel the world (water networks, electricity networks, etc.) to carry out technical interventions on the field. In this context, image recognition has the possibility of helping them in their daily tasks.
We quickly understood that there was one thing in common to all the use cases we address: a diagnosis can be made from a situation that we take a picture of. Thanks to AI, the diagnosis is instantaneous and reliable.
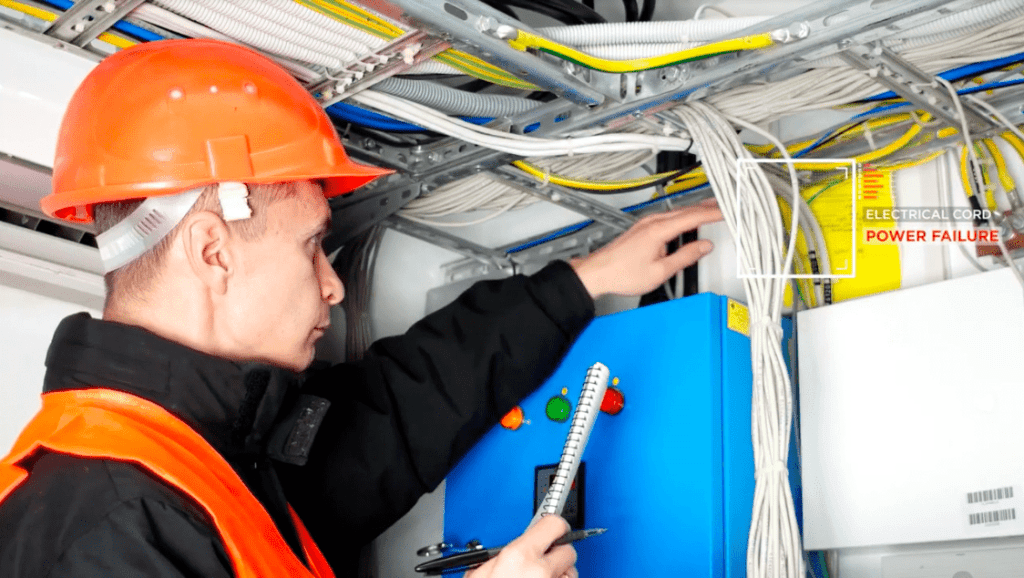
In the telecoms sector, there is an extremely concrete application case. Indeed, many individuals encounter problems when connecting equipment to optical fiber. In the industrial sector, there is a production defect among thousands or even millions of items produced. Conversely, in the world of field operations, operations are more fluctuating with subcontracting chains that imply gigantic failure rates. Typically, in telecoms a lot of interventions do not go to the end.
Our goal is therefore to help the technician to succeed in his intervention the first time. To do this, he takes a photo of his intervention at 5 to 10 key moments, and he will be able to receive feedback in real time, be able to correct the situation instantly if necessary and therefore succeed in his intervention. Moreover, the fact that the quality control, before a posteriori, is carried out at the time of the intervention, makes it possible to systematize the intervention, and to exercise better quality control. As this use case is very widespread and central for a number of service companies, we have chosen to focus on this issue.
How to simplify the daily life of data scientists when they work on AI topics?
In fact, Deepomatic is an extremely valuable additional information source for data science. It is an efficient platform for processing images and videos of the terrain to extract precise information.
If I take the example of the SNCF, the rails represent an asset and constitute a network. Today trains are equipped with cameras and as they pass the rails we are able to visualize the condition of the rails and map the wear condition of those rails. It is also possible to see the places where the rails deteriorate more quickly and therefore to act in a preventive manner.
These image and video content will in short increase the amount of information available to Data Scientists, more quickly. And we work with Data Scientists to solve real problems.
Deepomatic is also a tool that allows you to easily iterate over your dataset with fixed models. This is indeed one of the pain points often found among Data Scientists. Thanks to Deepomatic, data scientists can easily optimize their datasets.
In your opinion, image recognition can be applied to other areas, which ones? and by 2030 how do you see this sector evolving?
My bet is to say that today the application cases that we see in general are obvious. I am obviously thinking of quality control in factories, identification of people (smartphones, website …), anti-terrorist facial recognition, autonomous vehicles … Some cases we hear about are not yet a reality today. Indeed, we are currently in a phase of simplifying everyday problems, a phase of automating simple tasks. But by 2030, some promises may be kept.
Today, we notice that there is not just one application case, there are already thousands. On the other hand, only very few cases are actually in production. For example, in medicine we are already faced with endless possibilities. The constraint is that it takes almost absolute precision to provide real assistance to doctors. You have to work a lot on a very specific case to arrive at a precise and automated diagnosis. By 2030, we will break down the major fields of application mentioned above (robot movement capacity, quality control, understanding of behavior).
In addition, we can predict the arrival of a huge number of small applications that will simplify our lives, precisely because production costs will have decreased. We are already seeing it at our scale, especially in precision farming, for example.
Today, the observation is that image recognition is not sufficiently democratized and still too expensive to execute the thousands of cases already identified. The key, in my opinion, is to tame this technology, through tools like Deepomatic precisely, to lower production costs and allow large-scale deployment. This will increase the ROI of bringing these projects into production. We will also have a better understanding of how these projects are integrated, so that operational benefits are tangible and acted upon.
Image recognition poses significant ethical questions, how does Deepomatic fit into this vision?
At Deepomatic, we have made the choice to handle the thousands of application cases that were not really concerned with ethical issues. The use cases most affected by ethical questions are facial recognition and autonomous vehicles. If you do not cover these topics, then you are less likely to be confronted with these questions. Our mission is to support the technician in his daily tasks. There are always risks as to how to implement this technology so as not to sanction it. Nonetheless, we have two major workhorses from a CSR perspective.
First, some ethical topics that are of particular interest to us are optimization topics that lead to job transformation. We are part of an important globalization process which can be violent in times of crisis, and we must know how to accompany these changes so that they are as fluid as possible.
Second, another topic of particular concern to us is the environmental impact of artificial intelligence. The question is how to minimize this impact. Today we have the ability to know very much how much CO2 we are generating. We have created a very specific model in this regard. In addition, we have helped to absorb as much CO2 as we emitted in order to be carbon neutral. We are also able to communicate the environmental impact of most of our use cases to our customers.
What about the risks associated with facial recognition?
Personally, I find these technologies very scary, I don’t want people to know where I am all the time. It is absolutely necessary to have a deep reflection on the subject. These technologies are very powerful and can be used for toxic purposes that go against the principles of democracy, of freedom that we know today. And this is partly why we have chosen to turn to civilian applications.
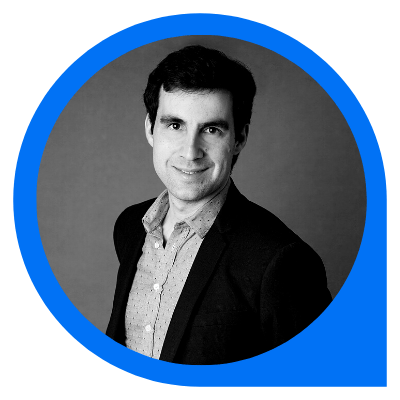
CEO and President of Deepomatic, Augustin Marty studied economics at École des Ponts in Paris and at UC Berkeley. He launched a computer tablet company in China, before joining industrial construction group Vinci, as a sales engineer for 2 years.
Augustin drives Deepomatic’s vision to transform computer vision deployment, staying focused on delivering value to our clients, and go-to-market timing. Capitalizing on his experience at Vinci, Augustin understands the challenges of large industrial groups and the complexity of their decision-making process.